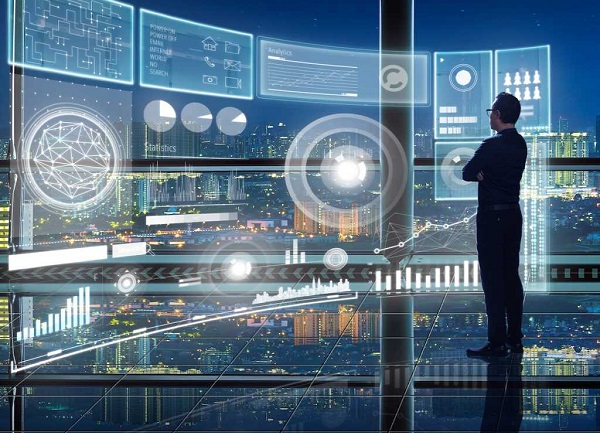
Sponsored by Dell Technologies and Intel®
By Nigel Steyn, Sales CTO, Computer Vision Edge IoT, Dell Technologies
Years ago, computer vision was just a clever science fiction innovation, but now it’s a reality that is quickly becoming a part of the enterprise landscape.
In essence, computer vision uses AI and machine learning to make sense of digital video, images, audio and even biometric data, and provides insights and conclusions. With data gathered from both cameras and edge based IoT sensors dotted around a building, campus or an entire city, for example, computer vision technology applies algorithmic models to learn about visual data and turn it into information for decision making.
According to Forrester, 80% of organizations expect the number of AI use cases to increase in the next two years.[1] These data-driven organizations are implementing computer vision to improve the customer experience, gain operational efficiencies and ultimately drive new revenue streams. Indeed, IDC says that organizations that are considered data analytics innovators are two times more profitable than their peers.[2]
It all makes sense. Reliable, accessible data helps business and operational leaders make better decisions, strengthens security, gives companies a competitive edge and can transform how organizations deliver products and services.
The missing link: a real-time federated approach
Video intelligence is not new. Most companies, public spaces and even today’s schools are outfitted with video camera systems. What’s standing in the way of translating video into real-time actionable insights, however, is managing the volume, velocity and variety of data, as well as connecting the dots on that data.
While common approaches to data curation and analytics send data to a central platform for processing, doing so can create tremendous amounts of traffic across a network. There’s also latency involved with large data flows traveling back and forth, which is a significant issue if time-sensitive decisions must be made based on the data.
In order to overcome these challenges, some organizations are implementing real-time federated learning models (Figure 1) which allow for the data to be more efficiently processed and stored, for the most part, at the network edge. The idea is to build algorithmic models on a central server, which is often in the cloud, and keep data at the edge where it’s used. Send a model to the edge, train it with the local data, and then send back only the results to the central server for aggregation. In turn, the central model can be improved and sent back to the edge for enhanced local inferencing.
Figure 1. A Federated Learning Model: The flow of information in a federated model keeps data in place from each edge device while enabling secure data sharing of metadata, results and models across users, devices, data centers, and the cloud.
Today’s visual data models are also designed with typical end users – at the edge – in mind. Having data scientists on hand to perform analyses has largely been replaced with standardized models and drag-and-drop workflows for a “create your own” analytics package. An analytics model marketplace has emerged where companies that are investing in computer vision technology but don’t have in-house AI or data science expertise can incorporate models into their operations.
There are significant advantages to the real-time federated approach:
• You ingest visual data once and can allow multiple applications access to the information.
• The model that remains on the central server is not tied to specific data; models can be shared with others without security risks.
• Because training takes place at the edge, network costs are lower and there’s no need to maintain a centralized data lake.
• The data marketplace democratizes computer vision for the masses, enabling the sharing and monetization of pre-trained models aligned with specific use cases.
Computer vision’s impact today
Computer vision touches our everyday lives. You probably use computer vision without realizing it. Does your smartphone have facial recognition that lets you unlock the device or authenticate to online banking sites? That’s computer vision. Consider modern cars with embedded cameras. Visual data used from the cameras as well as other sensors gives modern cars the ability to parallel park themselves. Adaptive cruise control regulates a car’s speed while leaving a safe gap between itself and other vehicles, and the accident avoidance system applies the brakes when a car is too close to an object in front of it.
From a business perspective, you can find applications of computer vision across nearly every vertical – retail, transportation, healthcare, manufacturing and energy, to name a few. And you can apply computer vision across those verticals to achieve substantial outcomes, such as personal and facility safety, improved customer experience, operational efficiencies, sustainability and revenue generation. With good visual data and constantly improved models, airports can be run more efficiently, stadium customer service can be customized for each spectator and the healthcare industry can detect tumors more accurately and quickly.
Where is computer vision heading?
To answer this question, let’s look at Figure 2, which depicts the maturity curve of analytics. Over the past few years, many organizations have moved from using Computer Vision as a means to retrieve just descriptive and diagnostic results to being able to be more predictive in their approach, where the models help to predict likely outcomes.
Dell
Figure 2. Analytics Maturity Curve
Data-driven organizations are now deploying prescriptive solutions that are driving tangible benefits, outcomes and near real-time actionable insights. As these solutions become more adaptive, they will bring dramatic improvements to the performance of systems and automated processes, as well as decision making. And that’s when a real-time continuous improvement loop will be possible, where a model is constantly retraining itself. The later stages of the analytics maturity curve will see processes occur with little or no human interaction or decision making, with resources reaching their destination proactively.
The computer-vision-enabled car will automatically integrate with smart city traffic control systems to avoid and help alleviate traffic congestion, and it will connect with parking information to be guided to the most efficient spot based on its destination. With real-time computing at the edge and an updated model being pushed to the car, your car will “know” how to drive safely whether it’s in a senior community neighborhood or on a high-speed freeway.
Moving forward with a computer vision strategy
Adopting a computer vision solution requires proper testing and validation. Anyone can run compatibility tests and validate an application on a platform in a silo. The trick is to validate multiple applications that are working together on a platform at scale, which support the “ingest once, work on many” concept, and that span the full solution architecture from edge to core to cloud.
A computer vision model design should be validated to ensure the models work properly with specific real-world computer vision applications. Validated design solutions are tested and optimized for each use case. They’re also right-sized to the environment in which they will run, which takes the guesswork out of how much storage, compute and other specs you’ll need for proper purchasing. Opting to conduct real-world testing in-house can put a strain on resources but there are third-party options available. Dell Technologies, for example, offers lab-validated solutions from a curated pool of more than a hundred technology, AI and service partners.
Where validation addresses the technical elements, computer vision is also about the business. Each organization looking to adopt computer vision technology or bring their current implementation to the next level should focus on the insights they want to gain in order to run their business and improve processes. With computing done at the edge, previous boundaries and limitations are lifted. We can now deliver better quality insights, faster and at scale, so organizations should first focus on the outcomes they want to achieve in the areas of safety, customer experience, operational efficiencies, sustainability and generating additional revenue
Computer vision technology provides deeper insights than you can get just from data. Now you can complement that data with visual information, making the data much richer and more useful, and maintain a competitive edge in this new era of how organizations operate and serve customers.
For more information:
• Read the Solution Briefs
o Delivering game-changing, actionable intelligence with AI-Driven Computer Vison
o A federated learning platform for real-time Artificial Intelligence
• Contact a Computer Vision expert
• www.delltechnologies.com/safetyandsecurity
• Stay tuned: This is the first article of a series depicting how computer vision is impacting key industries. Be on the lookout for future articles from Dell Technologies on this topic.
________________________________________
[1] Forrester report: “Overcome Obstacles To Get To AI At Scale,” January, 2020. https://www.ibm.com/downloads/cas/VBMPEQLN.
[2] Computer Vision 030421 pdf. IDC report: “The Data-Forward Enterprise: How to Maximize Data Leverage for Better Business Outcomes,” May, 2020. DOC #US46264420. https://bit.ly/3BDaOvh